Here at Hark, our mission statement is to:
Improve efficiency, maximise yield, and reduce waste.
Applying this to commercial energy consumption is vital, as energy costs and global greenhouse gas emissions continue to rise.
The effects are becoming more prominent, through natural disasters such as Storm Ciara and the Australian Bush Fires. Global greenhouse gas emissions must be eliminated within thirty years or else the world will face catastrophic consequences, according to a 2018 intergovernmental report. Despite this overwhelming evidence, emissions are continuing to rise.
Almost every action within modern society has an impact on the environment, meaning there are many chances to get involved. From reducing greenhouse gas emissions to limiting single-use plastics and developing emergency natural disaster plans, there are opportunities for all businesses and individuals to make a difference.
Recently, Machine Learning (ML) has gained recognition as a powerful tool, capable of solving a wide variety of problems. But how can these tools be used to tackle Climate Change? This question was investigated by numerous AI and ML researchers, including Andrew Ng, David Rolnick and Yoshua Bengio, who published a paper on the subject. You can find more information on their project website. The paper looks at many different areas of society, and comments on which ML methods and techniques are applicable. Paul Strobel’s blog post also does an excellent job of summarising the findings.
This blog post will be looking at how machine learning can be used to tackle Climate Change within Electricity Systems, and Buildings & Cities. This is an adaptation of a talk I recently gave at LeedsML, which is a group for data scientists and machine learning engineers looking to share knowledge.
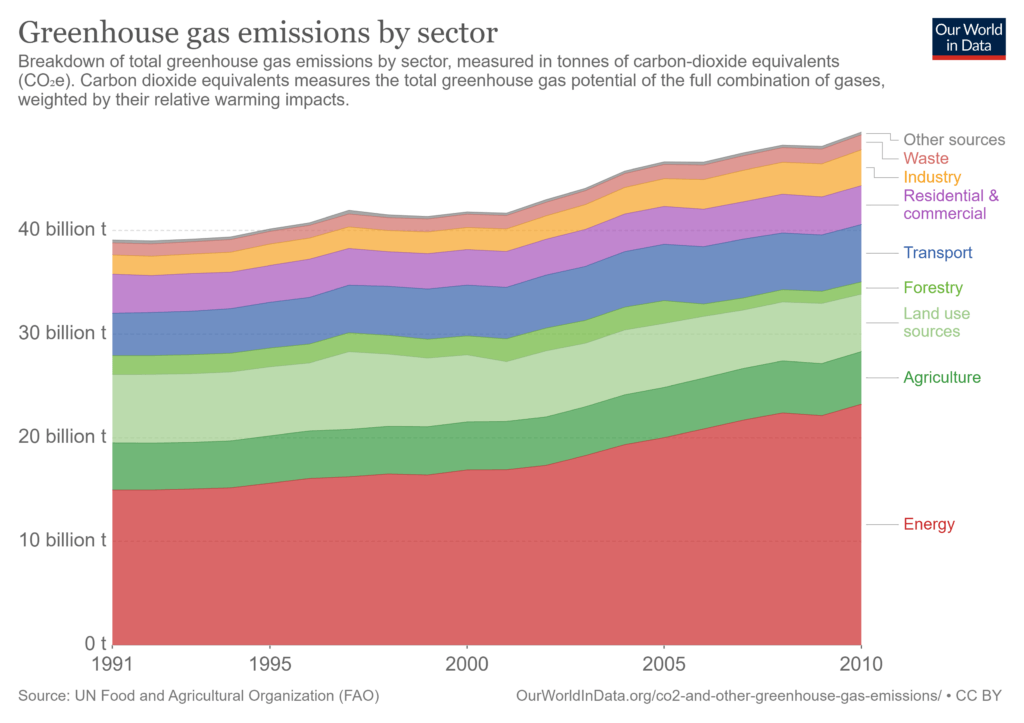
Electricity Systems
Electricity systems refer to anything related to the production of electricity. This includes nuclear and fossil fuel power stations, the global power grid, and renewable energy technology. These systems are responsible for about a quarter of human-caused greenhouse gas emissions. As buildings, transportation, and other sectors seek to replace GHG-emitting fuels, demand for low-carbon electricity will grow. ML can help drive the change towards smart grids, allowing for optimisation of power demand and usage.
Forecasting Power Production and Demand
Improving energy forecasts can directly reduce emissions from this sector. Renewable energy production is highly variable, depending on inconsistent wind conditions and solar radiation.
To pick up the slack during production shortages, energy grid operators use standby fossil fuel plants called spinning reserve. In the future, this slack may be managed by energy storage solutions like batteries and hydropower. Until energy storage is widespread, Machine Learning allows for better short term wind (using SVM and Genetic Algorithms) and solar (using SVM, Regression Trees, Random Forests) power generation forecasts and total power demand (using Regression, Neural Networks). In turn, this helps sever our reliance on polluting fossil fuels. Longer-term forecasts can also assist in deciding when and where renewable power plants should be built.
Looking at solar power, grid operators can make better predictions if they know their operating area’s panel capacity. Using machine learning and satellite data, rooftop solar panels can be visually detected, giving estimates of capacity (using Random Forests). We can also use machine learning techniques on historical data around the power generation of panels to predict faults (using Regression). This can be used to inform the affected customers and alter short term solar production forecasts.
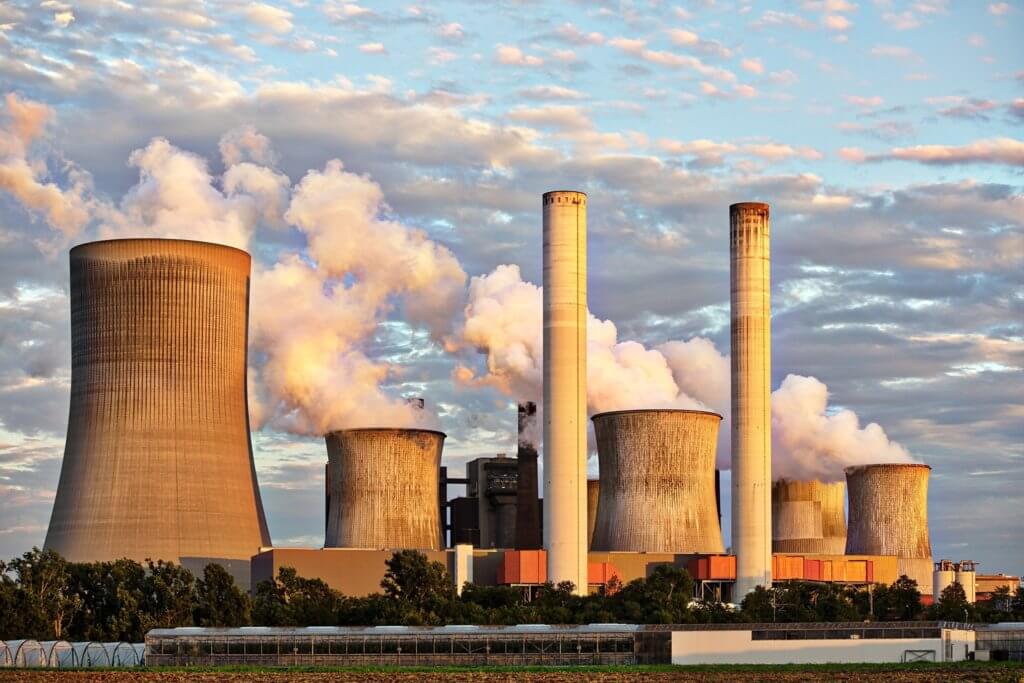
Reducing Fossil Fuels Lost in Transit
We can also reduce fossil fuels lost during transport. In the case of Methane, its release into the atmosphere should be avoided as its greenhouse effect is 30 times more potent than CO2.
Looking at a natural gas pipeline, ML can be used to detect a leak (using SVM). This is offered by companies such as Project Bluefield Technologies, using both on-site sensors and satellite sensors to learn the spectral signature for methane, which is reflected off the ground if a leak is occurring. Relevant teams can then be despatched to the affected area.
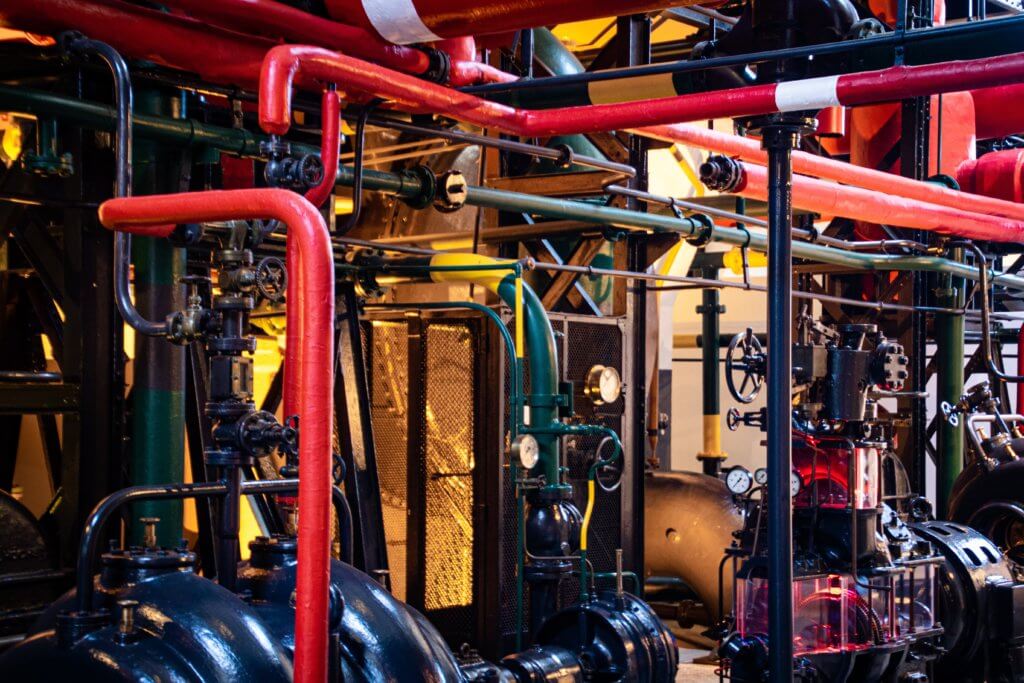
Emissions Modelling and Visibility
It’s important that we are aware of the amount of emissions being released at any given time. Industrial and domestic consumers cannot make climate-friendly decisions if they don’t know what’s going on. Using ML, we can model current and future emissions of electricity. This can be used to inform people when consumption is most environmentally friendly. For example, households running their washer-dryer when there is excess wind power on the grid. Below are some websites that use various techniques such as Ensemble Models and Regression to provide insight into live emissions.
Live European Energy Consumption and Production – electricityMap
US Based Clean Energy Reporting – WattTime
UK Carbon Intensity API – Carbon Intensity
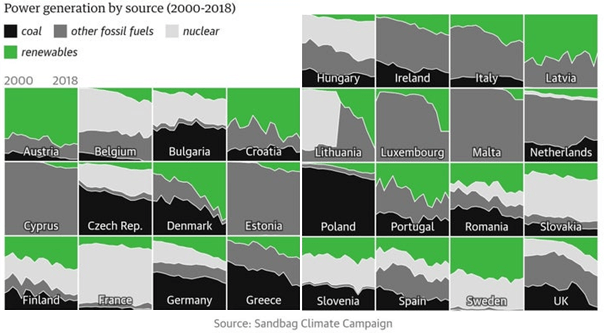
Buildings and Cities
A quarter of global energy-related emissions is caused by the power consumption in buildings. There is huge potential in this sector, with many easy to implement fixes, that are capable of both reducing emissions and saving costs.
Enabling Smart Buildings
Machine Learning can reduce energy usage by learning and recognising system usage patterns (using Reinforcement Learning and Decision Trees). This also helps to integrate variable power low carbon mentioned earlier. This allows for more flexible consumption and communication between the grid and buildings, lowering costs and emissions. Buildings capable of this are referred to as Smart Buildings.
Heating, ventilation and air conditioning (HVAC) is notoriously inefficient and responsible for more than half of energy consumption within buildings. By looking at usage, occupancy and weather patterns, Smart Buildings can reduce HVAC energy consumption.
Hark is currently solving these problems for the Bright Building, a 70,000sq ft office in Manchester. Here we manage a Tesla battery pack that’s capable of taking the entire building off grid. By analysing external sensor data, we can use stored power at peak time, and charge the batteries during off peak times, getting a better price per kWh.
Smart buildings can also recognise leaks in refrigerants from devices like air conditioners. Commonly found in refrigerants are HFCs, which have a 9000x greater global warming impact than CO2. Despite HFCs being phased out since January 2019, older devices that still contain HFCs will persist. Predictive maintenance using ML can identify these leaks (using Bayesian Networks) much faster than traditional methods, and even before they happen.
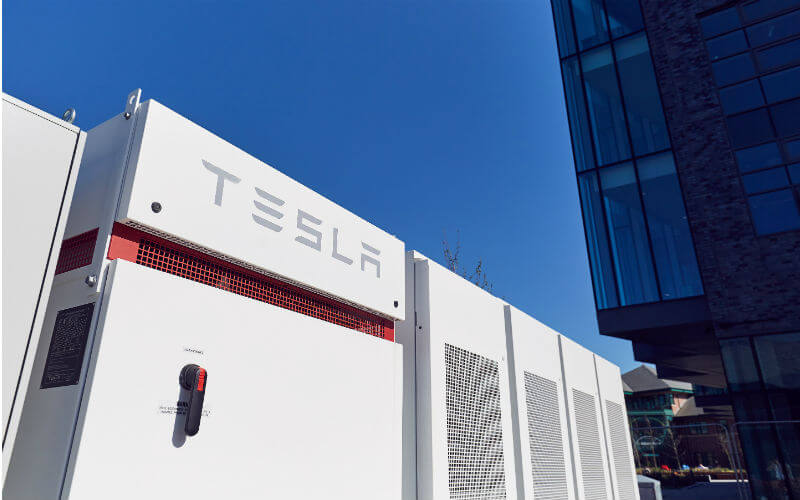
Enabling Smart Cities
Cities are collections of global resources, channeled into a small space. Local governments have a great opportunity to tackle Climate Change, due to the wide variety of issues they handle, including water, waste, and energy. Smart Cities can collect relevant data to help the government make informed decisions.
For example, the city of Los Angeles passed a regulation that requires vehicle-sharing companies (e.g. Lime and Bird scooter sharing services) to provide data on location, use and condition of all vehicles via an open-source API. This can be used to help shape policy around public transport and access routes.
Conversely, Machine Learning can also be used where there is a lack of data. Currently, many Smart City projects are conducted in the wealthier global north, despite that the largest Climate Change mitigation opportunities are in the global south. Using clustering, cities with similar climate-relevant factors can be grouped, allowing for Climate Change solutions to be transferred from city to city.
Conclusion
It’s vital that we go above and beyond the current climate change policies and pledges. As it stands, we are on track for a global temperature change of +~3.4⁰C. This will bring with it an increase in extreme weather and other unforeseen consequences. However, pathways to a global temperature change of +~2⁰C do exist, and through working together, are achievable. As seen above, it will require many solutions to many problems, as there is no magic bullet. However, this does present plenty of opportunities for businesses and individuals to really make a difference. For further investigation, Our World In Data has some eye-opening visualisations to help understand where we are in the fight vs Climate Change.
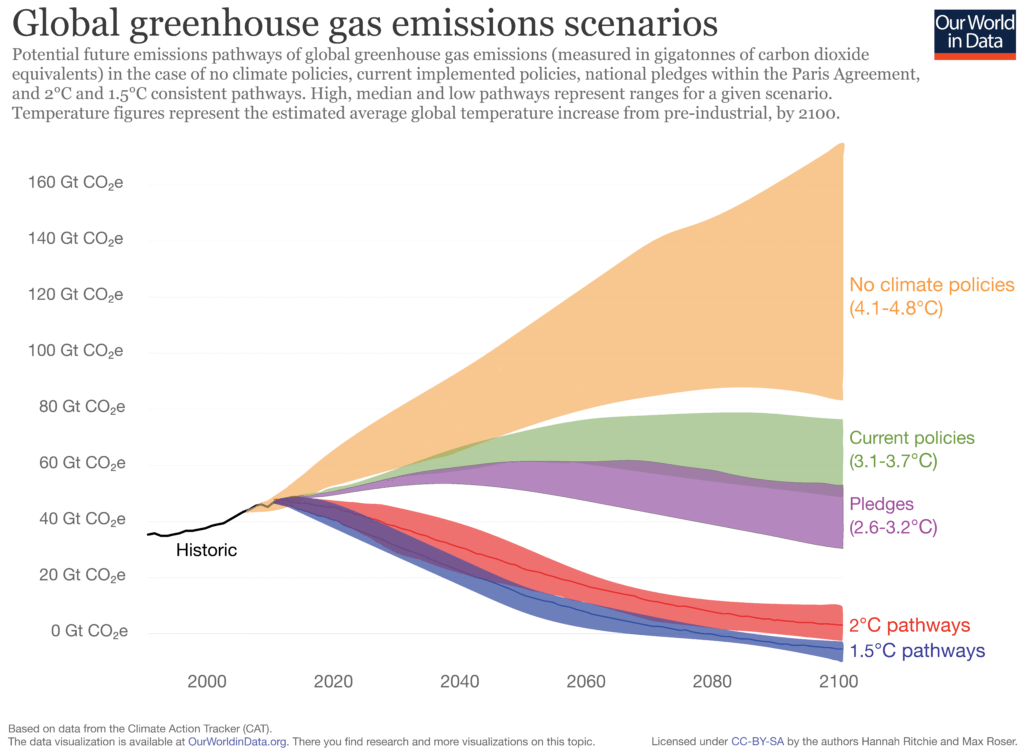